Oseyomon John Aigbokhan, Oludare Hakeem Adedeji, Abiodun Olusegun Oladoye, John Adebayo Oyedepo
ABSTRACT. In this study, the spatiotemporal dynamics of the urban environment and thermal environment of Benin City are analysed. The maximum likelihood algorithm for land use and land cover (LULC) analysis was used to categorise Landsat images. The relative transfer equation (RTE) and land surface emissivity (LSE) approaches were used to retrieve the land surface temperature (LST), whereas the Cellular Automata-Markov (CA-Markov) algorithm was used to forecast the LULC for 2030. The findings reveal evolving LULC patterns over time. Built-up areas made up 19.66% of the total area in 1990, bare ground made up 9.25%, and vegetation made up 71.08%. Built-up areas reached 23.40% in 2000, bare land reached 12%, and the vegetation cover dropped to 64.16%. In 2010, there was an increase in the proportion of built-up areas to 44.38%, the proportion of bare land increased to 22.20%, and the proportion of vegetation decreased to 33.42%. Built-up areas reached 61.79% in 2020, compared to 22.29% for bare land and 61.79% for vegetation. Regarding the relationship between the fractional vegetation cover (FVC) and LST, for the years 1992, 2002, 2012, and 2022, R2 is equal to 0.87097, 0.84598, 0.83957, and 0.71838, respectively. Conversely, for the LST and the normalised difference built-up index (NDBI), the R2 values were 0.5975, 0.73876, 0.86615, and 0.90368 for 1992, 2002, 2012, and 2022 respectively. In conclusion, this study evaluates Benin City’s metropolitan setting and thermal environment. According to the LULC study, there are more built-up areas and less vegetation. The impact of the changing land cover on urban thermal features is shown through correlation analysis, which links more built-up regions to higher LSTs. These results can support urban design efforts to lessen the effects of climate change. Examining the distribution of the LST and its associations with particular land cover types was the major goal of this study. Future research will undoubtedly use this study as a useful reference when modelling urban terrain and temperature variations.
Keywords: algorithm; concentric zone; emissivity; zonal statistics.
Cite
ALSE and ACS Style
Aigbokhan, O.J.; Adedeji, O.H.; Oladoye, A.O.; Oyedepo, J.A. Dynamics of urban landscape and its thermal interactions with selected land cover types: a case of Benin City, Nigeria. Journal of Applied Life Sciences and Environment 2023, 56 (2), 245-272. https://doi.org/10.46909/alse-562099
AMA Style
Aigbokhan OJ, Adedeji OH, Oladoye AO, Oyedepo JA. Dynamics of urban landscape and its thermal interactions with selected land cover types: a case of Benin City, Nigeria. Journal of Applied Life Sciences and Environment. 2023; 56 (2): 245-272.
https://doi.org/10.46909/alse-562099
Chicago/Turabian Style
Aigbokhan, Oseyomon John, Oludare Hakeem Adedeji, Abiodun Olusegun Oladoye, and John Adebayo Oyedepo. 2023. “Dynamics of urban landscape and its thermal interactions with selected land cover types: a case of Benin City, Nigeria” Journal of Applied Life Sciences and Environment 56, no. 2: 245-272.
https://doi.org/10.46909/alse-562099
View full article (HTML)
Dynamics of Urban Landscape and Its Thermal Interactions with Selected Land Cover Types: A Case of Benin City, Nigeria
Oseyomon John AIGBOKHAN1*, Oludare Hakeem ADEDEJI2, Abiodun Olusegun OLADOYE3 and John Adebayo OYEDEPO2
1Department of Environmental Modeling and Biometrics, Forestry Research Institute of Nigeria
2Department of Environmental Management and Toxicology, College of Environmental Resources Management, Federal University of Agriculture, Abeokuta; email: hakeemdare1222@yahoo.com; oyedepoja@funnab.edu.ng
3Department of Forestry and Wildlife Management, College of Environmental Resources Management, Federal University of Agriculture, Abeokuta; email: segun11us@yahoo.com
*Correspondence: oseyomon255@gmail.com
Received: Jun. 13, 2023. Revised: Jul. 28, 2023. Accepted: Jul. 30, 2023. Published online: Sep. 11, 2023
ABSTRACT. In this study, the spatiotemporal dynamics of the urban environment and thermal environment of Benin City are analysed. The maximum likelihood algorithm for land use and land cover (LULC) analysis was used to categorise Landsat images. The relative transfer equation (RTE) and land surface emissivity (LSE) approaches were used to retrieve the land surface temperature (LST), whereas the Cellular Automata-Markov (CA-Markov) algorithm was used to forecast the LULC for 2030. The findings reveal evolving LULC patterns over time. Built-up areas made up 19.66% of the total area in 1990, bare ground made up 9.25%, and vegetation made up 71.08%. Built-up areas reached 23.40% in 2000, bare land reached 12%, and the vegetation cover dropped to 64.16%. In 2010, there was an increase in the proportion of built-up areas to 44.38%, the proportion of bare land increased to 22.20%, and the proportion of vegetation decreased to 33.42%. Built-up areas reached 61.79% in 2020, compared to 22.29% for bare land and 61.79% for vegetation. Regarding the relationship between the fractional vegetation cover (FVC) and LST, for the years 1992, 2002, 2012, and 2022, R2 is equal to 0.87097, 0.84598, 0.83957, and 0.71838, respectively. Conversely, for the LST and the normalised difference built-up index (NDBI), the R2 values were 0.5975, 0.73876, 0.86615, and 0.90368 for 1992, 2002, 2012, and 2022 respectively. In conclusion, this study evaluates Benin City’s metropolitan setting and thermal environment. According to the LULC study, there are more built-up areas and less vegetation. The impact of the changing land cover on urban thermal features is shown through correlation analysis, which links more built-up regions to higher LSTs. These results can support urban design efforts to lessen the effects of climate change. Examining the distribution of the LST and its associations with particular land cover types was the major goal of this study. Future research will undoubtedly use this study as a useful reference when modelling urban terrain and temperature variations.
Keywords: algorithm; concentric zone; emissivity; zonal statistics.
INTRODUCTION
Population growth has been concentrated in metropolitan regions due to urbanisation, which has turned these areas into hubs of growth and economic activity (Bottalico et al., 2016). The loss of important ecosystems, increasing greenhouse gas emissions, landscape fragmentation, biodiversity loss, urban heat islands, and vulnerability to climate change are all consequences of this fast urban growth (Norton et al., 2015). Urban planning has gained popularity, particularly in industrialised countries, with a focus on constructing compact, dense cities, sustainable transportation, and improving liveability for both current and future generations (Demuzere et al., 2014). Important ecosystems are also destroyed and fragmented as a result of urbanisation, which has an effect on urban biodiversity and changes how cities interact with the environment (Wu, 2014). Cities are improving their infrastructure to solve these issues, with an emphasis on creating urban green and blue infrastructures to lessen the urban heat island effect (Connop et al., 2016). Natural vegetation, parks, private gardens, street trees, green roofs, and wetlands are just a few examples of urban green infrastructure, which is essential for delivering ecological, social, and economic advantages while reducing the negative effects of climate change (Pakzad and Osmond, 2016). The use of urban cool islands (UCIs) to minimise the urban heat island effect in Benin City has received very little investigation, despite prior studies exhaustively examining urban heat islands in Benin City (Agheyisi and Andrew, 2013; Efe and Eyefia, 2014; Dirisu et al., 2015; Igun, 2017). The collection of geographical information, such as archival satellite data, has been made possible by geospatial technologies; these data can provide insights into microclimatic phenomena like heat islands and prospective methods for enhancing human comfort (Blanusa et al., 2013; Skoulika et al., 2014; Okhakhu, 2016). Nigeria’s rapid, haphazard, and unregulated urbanisation makes it essential to precisely measure the emissivity of urban surfaces since cities can contain a variety of materials with an emissivity below 1 (Sobrino et al., 2012).
In many studies of the impact of urban heat islands, emissivity classification across urban areas was used (Imhoff et al., 2010; Myint et al., 2011). It has been acknowledged that urban green infrastructure, such as networks of public open spaces, urban tree canopies, wetlands, and green walls, has a favourable effect on the urban environment; it can supply services such as climate adaptation, urban resilience and sustainable development (Ashley et al., 2011; Foster et al., 2011; Norton et al., 2015). To meet the social and psychological demands of people living in cities, green areas should be available and well-maintained (Haq, 2011). They help cities look better, use less energy to cool the air, and generally cool down urban areas (Sadeghian and Vardanyan, 2013; Parker and Zingoni de Baro, 2019). The building density, urbanisation, and changes in land use and land cover all have an impact on the thermal properties of urban areas, which in turn affect the microclimate (Alavipanah et al., 2015; Okhakhu, 2016). The UCI effect is characterised by lower temperatures due to shade and other causes, whereas the urban heat island effect is principally produced by the significant heat created by urban structures, resulting in the absorption and re-radiation of solar energy (Hathway and Sharples, 2012; Nastaran, 2014). Green spaces, sometimes referred to as UCIs or green-space cool islands (GCIs), have been acknowledged as a mitigating approach that can alleviate heat stress (Feyisa et al., 2014; Skoulika et al., 2014).
Land surface temperatures can be determined using remote sensing methods that employ satellite data from programmes like Landsat and ASTER, which have also been used to track changes in the land use and land cover over time (Takeuchi et al., 2010). Because of the reduction in greenery, the expansion of paved surfaces, and human heat outputs, the temperature distribution in metropolitan centres is frequently warmer than in surrounding suburban areas (Elsayed, 2009; Akbari, 2011; Giannaros and Melas, 2012; Senanayake et al., 2013). Prior research has concentrated on comprehending the urban heat island effect and investigating methods that can lessen its adverse consequences (Choi et al., 2012; Gago et al., 2013). To identify urban heat islands (UHIs) in Benin City, the Urban Thermal Field Variance Index (UTFVI) was used. This index is widely used to describe the UHI effect with more precision (Tomlinson et al., 2011). The notable effects of a high UTFVI include but are not limited to adverse impacts on the local wind, humidity, and air quality, a reduction in comfort, an increased mortality rate, and indirect economic loss (Sejati et al., 2019).
An effective machine learning approach for classifying the land use / land cover (LULC) in images is called the random forest (RF) method. To produce precise forecasts, RF combines the strengths of decision trees and ensemble learning. There are countless algorithms for classifying images, including support vector machines (SVMs) (Kamavisdar et al., 2013), maximum likelihood (ML) models (Otukei and Blaschke, 2010), deep learning (DL) models (Alzubaidi, 2021; Naushad et al., 2021), and fuzzy classification (FC) models (Badhe and Chang, 2016). Due to its superior effectiveness and accuracy, cheap computational cost, and small number of parameters, RF has emerged as one of the top methods for LULC mapping (Thanh Noi and Kappas, 2018; Naushad et al., 2021). The following research topics were addressed in this study: (a) What changes have occurred in the LULC of the study area? (b) How do the various land cover types in the study area relate to the urban temperature variability? Examining the distribution of the land surface temperature (LST) and its associations with particular land cover types is the major goal of this study. This study began with the consideration of numerous linked research articles. However, the major gap that this study is meant to fill is the gap that exists because past studies have not given enough attention to the thermal interactions between the urban landscape and particular types of land cover, with a focus on microclimatic characteristics.
MATERIALS AND METHODS
Benin City is situated between 6° 23′ 55″ and 6° 27′ 39″ N and 5° 36′ 18″ and 5° 44′ 31″ E in the Nigerian state of Edo (Aiyesanmi and Imoisi, 2011). It currently serves as Edo’s capital and was formerly the regional capital of the old Midwest. Figure 1 shows the location of the city, which spans three major local government areas: Oredo, Egor, and Ikpoba-Okha (Okhakhu, 2010). Benin City is located in the Benin Formation, a geological deposit from the Miocene to Pleistocene eras (Odemerho, 1988). Low-lying, undulating terrain with gentle slopes toward the Ogba Stream in the western section and the Ikpoba River in the eastern section make up the topography (Eseigbe and Ojeifo, 2012). Reddish soil made of lateralised clay sand and huge lateritic clay and sand layers make up the region’s geology (Akujieze, 2004). Tropical weather with distinct rainy and dry seasons is the norm in Benin City.
The dry season normally lasts from December to March, whereas the rainy season typically lasts from mid-April to mid-November. The city has a mean monthly temperature of 28°C (82.40°F), with a typical annual rainfall of 2000–2500 mm (Ezemonye and Emeribe, 2014). Although urban development has considerably decreased the amount of vegetation, the majority of Benin City’s vegetation consists of evergreen rainforest (Ezemonye and Emeribe, 2014). The city is renowned for its numerous mediaeval moats, which surround different districts. Primary, secondary, and tertiary sectors all contribute to the socio-economic activities of Benin City’s thriving economy.
Brass casting, wood carving, blacksmithing, general buying and selling, and transportation are examples of the economic activity that takes place in the city (Ezemonye and Emeribe, 2014; Onaiwu, 2021). The city is home to several markets, including Ikpoba Hill Market, Uselu Market, New Benin Market, and Oba Market.
This study analysed the study region over 30 years using Landsat satellite images from the Thematic Mapper (TM), Enhanced Thematic Mapper (ETM), Enhanced Thematic Mapper Plus (ETM+), and Operational Land Imager (OLI) sensors, which were downloaded from the US Geological Survey’s main website (USGS, 2022). Except for the thermal Infra-Red bands, the images from 1990, 2000, 2010, and 2020 were collected with pixel sizes of 30 m by 30 m.
The pixel sizes and specifications were obtained from Chander and Markham (2003). The US Geological Survey’s official website was used to retrieve the Shuttle Radar Topographic Mission (SRTM) data, which were also used in the study. All satellite images were captured during the dry season to ensure uniformity and minimise the cloud cover. The dry season was chosen due to the generally decreased cloud cover during this time. Additionally, the seasonality had no substantial impact on the study’s goals. Using the WGS-84 data, the Landsat images were georeferenced to the UTM zone 31 North projection. The dates, path/row, and specifications for the OLI, ETM+, and Landsat TM images are listed in Table 1. Although not all bands were directly relevant to this inquiry, Tables 2 and 3 list the bands, their bandwidths, and their resolutions. In addition to the Landsat images, a high-resolution geo-referenced dataset from Google Earth Imagery (GEI) was also employed, particularly in urban areas where land cover patterns are made up of a complex mosaic of various land uses. The study concentrated on examining how the environment changed and how the UCI phenomenon manifested during 1992, 2002, 2012, and 2022. This study used ArcGIS 10.4.1, QGIS 3.12, Idrisi Selva 17, R/RStudio 3.5.2, and a portable GPS, among other software programs and tools. Several activities, including making shapefiles, enhancing maps, and managing vector-related components, were completed using ArcGIS 10.4.1. For accuracy evaluation and image pre-processing, QGIS 3.12 was used. For the study’s geostatistical analysis, Idrisi Selva 17.0 was utilised.
Table 1
Satellite images and their acquisition dates
Satellite sensor |
Spatial resolution |
Acquisition years |
Time of day |
Path |
Row |
Source |
TM |
30 m × 30 m |
14-01-1992 |
02:43:16 |
189 |
56 |
USGS (2022) |
ETM |
30 m × 30 m |
07-02-2002 |
10:36:22 |
189 |
56 |
|
ETM+ |
30 m × 30 m |
03-02-2012 |
04:22:46 |
189 |
56 |
|
OLI_TIRS |
30 m × 30 m |
07-03-2022 |
09:51:06 |
189 |
56 |
|
Google Earth Imagery |
2.5 m × 2.5 m |
1992, 2002, 2012, and 2022 |
– |
– |
– |
Google Earth Pro |
SRTM image |
30 m × 30 m |
– |
– |
– |
– |
USGS (2022) |
We conducted a further statistical analysis using R/RStudio 3.5.2. The ground control points (GCPs) were gathered using a portable GPS device. The precise spatial referencing of the satellite images was made possible by these GCPs, which are essential to the georeferencing process. Overall, various software systems and tools were chosen based on their value and how well they fit this particular project’s needs.
Image Pre-processing
To eliminate atmospheric effects and obtain precise surface reflectance values, it is essential to atmospherically correct satellite photos. The dark object subtraction (DOS) technique is a frequently applied atmospheric correction algorithm. The DOS algorithm assumes that there are certain dark objects in an image that, in the absence of air scattering and absorption, should have a reflectance value near zero. A portion of this algorithm was utilised for image processing. In each of the k bands of imaging, the image elements (pixels) that make up a digital remotely sensed image are typically located at the intersection of each row i and each column j. The images used in this study were first converted to top-of-atmosphere (TOA) radiance data using Equation 1 (Giannini et al., 2015):
where:
= the spectral radiance at the sensor’s aperture [W/(m2 sr µm)],
QCAL = the quantised calibrated pixel value [DN],
QCALMIN = the minimum quantised calibrated pixel value corresponding to LMIN [DN],
QCALMAX = the maximum quantised calibrated pixel value corresponding to LMAX𝝀) [DN],
LMIN = the spectral at-sensor radiance that is scaled to QCALMIN [W/(m2 sr µm)], and
LMAX = the spectral at-sensor radiance that is scaled to QCALMAX [W/(m2 sr µm)].
The above expression does not consider the atmospheric effects, and therefore there is a need to convert the images from radiance to reflectance measures using Equation 2 (Giannini et al., 2015):
where:
the planetary TOA reflectance (unitless),
π the mathematical constant approximately equal to 3.14159 (unitless),
the spectral radiance at the sensor’s aperture [W/(m2 sr µm)],
the Earth-sun distance [astronomical units],
the mean exoatmospheric solar irradiance [W/(m2 sr µm)], and
θSZ = the solar zenith angle [degrees]. The cosine of this angle is equal to the sine of the sun elevation θSE. That is, θSZ = cos (90 – θSE).
Image Classification
In remote sensing, image classification is a key procedure that involves separating different groups or themes, such as different types of LULC, from unprocessed digital satellite data. It allows the recognition and classification of many properties that can be observed in satellite images, such as the urban land cover, plant types, structures, and mineral resources, as well as changes in these aspects (Lillesand and Kiefer, 2008). Because of their high-quality data, Landsat images were utilised in the classification method in this study (Tables 2 – 4). Landsat satellite images have been extensively used in a variety of remote sensing applications and offer useful information for analysing the land cover.
Image classification is a difficult and time-consuming procedure that calls for a thorough assessment of the best classification methodology to obtain a high accuracy. The supervised classification approach was used in this investigation. This technique, which is popular in image classification, automatically classifies pixels with comparable spectral values into certain land cover classes or themes. Landsat data were used to map the LULC in 1992, 2002, 2012, and 2022 (Table 1). A modified version of the LULC classification scheme of Mikias et al. (2017) was used to classify the images (Table 5). The ensemble learning approach is used by the RF classifier as a machine learning methodology. Breiman (2001) initially presented this technique (Rimal et al., 2017). The number of iterations for each attribute is counted when the classification is complete using the RF method. It is important to note that as the number of trees grows, the overall classification accuracy grows as well (Rimal et al., 2017) until convergence starts to occur without overfitting. Sub-pixel studies on the surface temperature rely heavily on vegetation indices such as the normalised difference vegetation index (NDVI), fractional vegetation cover (FVC), and normalised difference built-up index (NDBI), which were derived using mathematical methods.
Normalised Difference Vegetation Index
Using digitised Landsat data, Rouse et al. (1974) developed the NDVI as a spectral vegetation index (VI) to separate green vegetation from the brightness of its backdrop soil. The difference between the reflectance values of the near-infrared (NIR) and red bands and their total reflectance is used to determine the NDVI. Because it successfully minimises topographic impacts and offers a linear scale for measurement, this index is extensively utilized.
Table 2
Band properties of Landsat 5 TM
Band |
Name |
Bandwidth (μm) |
Resolution (m) |
1 |
Blue |
0.45–0.52 |
30 |
2 |
Green |
0.52–0.60 |
30 |
3 |
Red |
0.63–0.69 |
30 |
4 |
NIR |
0.76–0.90 |
30 |
5 |
MIR |
1.55–1.75 |
30 |
6 |
TIR-1 |
10.40–12.50 |
120 |
7 |
TIR-2 |
2.08–2.35 |
30 |
Source: Chander and Markham (2003)
Table 3
Band properties of Landsat 7 ETM+ (2010)
Band |
Name |
Bandwidth (μm) |
Resolution (m) |
1 |
Blue |
0.45–0.52 |
30 |
2 |
Green |
0.52–0.60 |
30 |
3 |
Red |
0.63–0.69 |
30 |
4 |
NIR |
0.85–0.88 |
30 |
5 |
SWIR-1 |
1.57–1.65 |
30 |
7 |
SWIR-2 |
2.09–2.35 |
30 |
6 |
Thermal |
10.40–12.50 |
60 |
8 |
Panchromatic |
0.52–0.90 |
15 |
Source: Ihlen (2019a)
Table 4
Band properties of Landsat 8 OLI/TIRS
Band |
Name |
Bandwidth (μm) |
Resolution (m) |
1 |
Coastal |
0.43–0.45 |
30 |
2 |
Blue |
0.45–0.51 |
30 |
3 |
Green |
0.53–0.59 |
30 |
4 |
Red |
0.64–0.67 |
30 |
5 |
NIR |
0.85–0.88 |
30 |
6 |
SWIR-1 |
1.57–1.65 |
30 |
7 |
SWIR-2 |
2.11–2.29 |
30 |
8 |
Panchromatic |
0.5–0.68 |
15 |
9 |
Cirrus |
0.36–0.38 |
30 |
10 |
TIRS 1 |
10.6–11.19 |
100 |
11 |
TIRS 2 |
11.5–21.51 |
100 |
Source: Ihlen (2019b)
Higher NDVI values correspond to healthier and denser vegetation; the NDVI a typical range of -1 to 1. Generally speaking, NDVI values above 0.2 are frequently linked to vegetation, and values below 0.2 are typically connected with non-vegetated environments like rock, soil, or man-made materials. An NDVI map can be produced using Equation 3:
where: = the near-infrared reflectance and the red reflectance.
Table 5
A land use / land cover classification scheme
Built-up area |
An urban region with impermeable pavement (roads, pavement, and other highways) and structures |
Vegetated area |
Green space (land that is partially or entirely covered by grass, trees, shrubs, or other vegetation). Green space consists of public parks, neighbourhood gardens, plantation and natural forests, greenways, green spaces, institutional grounds, cemeteries, and religious grounds. |
Bare land |
Remnant parcels, which are often tiny in size and frequently spherical in form and have not been developed in the past; parcels with physical restrictions, such as a high slope or flood danger, which are thus unusable; or reserve parcels maintained for future relocation and enlargement |
Source: Mikias et al. (2017)
Fractional Vegetation Cover
The FVC is the projected percentage of the overall study area that is vegetated (with roots, stems, and leaves) (Gitelson et al., 2002). The size of the photosynthetic area, the density of the vegetation, and the development trend of the vegetation are all partially captured by the FVC, according to Gao et al. (2017). Yan et al. (2017) claim that the FVC is often used as a crucial criterion for the balance and expansion of terrestrial ecosystems in research on climate change, soil, and hydrology. Equation 4 will be used to compute the FVC:
where: = the NDVI maximum and = the NDVI minimum.
Normalised Difference Built-up Index
The disparity in the range of reflection and absorption values in populated and uninhabited land sections was utilised to select the wavelengths of the Landsat images that were used. Bands 5, 6, and 7 of the Landsat 8 OLI, which correspond to Near Infra-Red (NIR), Mid Infra-Red (MIR), and Shortwave Infra-Red (SWIR) wavelengths, provide a high contrast level for identifying populated and uninhabited terrain areas. In bands 5, 6, and 7, there is also an inverse reflection ratio that can be used to distinguish built-up areas from other types of land cover (As-syakur et al., 2012). In band 4, vegetation reflects light well, whereas built-up areas reflect light poorly. In contrast, urban environments are easier to identify in band 5 than vegetated ones (Bouhennache et al., 2015). The built-up land cover picture can be produced using Equation 5, which is from the introduction to the NDBI provided by Zhao and Chen (2005). The formula for calculating the NDBI using Landsat 5 uses band 3 and band 4 (Equation 5 and Equation 6) (Chander and Markham, 2003):
where: = the red reflectance (band 3) and = the near infrared reflectance (band 4):
where: = the mid-infrared reflectance and the near-infrared reflectance.
The index was created based on the unique spectral response of built-up areas, which exhibit more reflectance in the MIR wavelength range than in the NIR wavelength range.
The Operation of the Maximum Likelihood Classifier
The maximum likelihood (ML) method is one of the most used supervised classification methods for remote sensing data. The possibility that a pixel belongs to a certain class serves as the basis of this approach. The basic theory assumes that (i) these probabilities are equal for all classes and that (ii) the input bands have normal distributions. Using a normal distribution assumption for each input band, the ML classification approach for remote sensing tends to overclassify signatures with large values in the covariance matrix. It makes use of the spectral distance method, which measures the separation between a candidate pixel’s measurement vector and the mean vector for each class signature. Although ML classification is computationally efficient, it may misclassify pixels since it does not take class diversity into account. The posterior distribution P(i|) that reflects the likelihood of a pixel belonging to a certain class is determined by the Bayes theorem, which forms the basis of this method (Asmala and Shaun, 2012) (Equation 7):
where:
P(ω|i) = the likelihood function,
P(i) = the a priori information, i.e. the probability that class i occurs in the study area, and
P(ω) = the probability that ω is observed, which can be written as Equation 8:
where:
M = the number of classes.
P(ω) is often treated as a normalisation constant to ensure that sums to 1 (Asmala and Shaun, 2012).
Each class in the ML classification has an area in multispectral space where its discriminant function is bigger than those of all other classes. Decision borders split these class areas, and the decision boundary between classes i and j occurs where Equation 9:
Accuracy Assessment
Accuracy evaluation is an important part of mapping remote sensing data since it reveals potential classification errors that can arise. Common mistakes in image classification include errors of omission and commission. The confusion matrix, commonly referred to as the error matrix, is a popular technique for evaluating the classification accuracy. At each sample unit, a comparison is made between the reference data and the classified map, with the reference data being represented by columns and the classified map being represented by rows. The matrix makes it easier to see errors of commission and omission. In this study, 400 GCPs were randomly chosen for each year, and the accuracy of all classifications was assessed using the Kappa coefficient. The Kappa coefficient and the error matrix can be used to assess the correctness of the thematic maps produced by classification techniques (Equation 10) (Olofssona et al., 2013):
where:
KAPPA = the Kappa index,
k = the number of matrix files,
Xii = the observation number in row i and column i (along the diagonal),
= the total marginal for row i and column i, respectively, and
N = the total number of observations.
Four hundred sample points are used in the accuracy assessment. The number of sample points was calculated using the ‘Taro Yamane formula’ (Adam, 2020). The ERDAS Imagine 2014 accuracy evaluation technique was used to obtain the accuracy rating. The producer’s accuracy (Pa), user’s accuracy (Ua), Kappa statistics (K), and overall accuracy (A) were determined from the classified images for the years 1992, 2002, 2012, and 2022 (Equation 11):
where:
n = the sample size,
N = the finite population (i.e. the size of the area = 75266 ha), and
e = the level of significance (0.05).
To distribute the 400 points for the accuracy assessment, this study used a stratified random sample technique; this design was chosen since it is useful for analysing change maps (Olofssona et al., 2013). The stratified reference sample points were created in the ERDAS Imagine environment and placed on the reference image for the accuracy evaluation. The tool automatically generates the error matrix, accuracy totals, and Kappa statistics to assess the accuracy.
Temperature Retrieval from Satellite Thermal Bands for the Study Period
The retrieval of the LST was carried out using the following four phases.
Conversion from Digital Number to Radiance
The conversion from a digital number to a radiance value was performed as follows (Equation 12):
where: = the TOAr (TOA radiance), i.e., the radiance measured by the sensor, = the band-specific multiplicative value, = the band-specific additive value and = the quantised and calibrated value.
Conversion from Radiance to Brightness Temperature
The satellite temperature in Celsius was calculated using Equation 13 (Agbor and Makinde, 2018):
where:
Bt = the temperature in degrees Celsius,
= the TOA spectral radiance
K1 and K2 = the thermal conversion constants from the metadata.
Estimation of Land Surface Emissivity
For a precise LST estimate, the land surface emissivity (LSE) is required. The LSE is a proportionality factor that scales the black body radiance (Ugur and Gordana, 2016) to quantify the emitted radiance and the ability to transmit thermal energy from the surface into the atmosphere. It was calculated using Equation 14:
where: is the vegetation proportion obtained from Equation 15:
Land Surface Temperature Extraction
The LST was derived from TM band 6 and OLI band 10 using an emissivity-corrected model (Equation 16):
where:
= the wavelength of the emitted radiance,
ρ = h×c/(1.438 ×10-2 m K),
σ = the Boltzmann constant (1.38 ×10-23 J/K),
h = Planck’s constant (6.626×10-34 J s),
c = the velocity of light (2.998 ×108 m/s) and
Ɛ = the emissivity (Lillesand and Kiefer, 2008)
The Urban Thermal Field Variance Index
The urban thermal field variance index (UTFVI), which was used in Equation 17 can be used to describe the UHI effect objectively. The UTFVI was computed in the ArcGIS environment using a raster calculator to quantitatively reflect the UHI effect:
where: = the LST and = the mean LST of the area.
The study area was further classified into six different regions based on UTFVI scores (Singh and Singh, 2017).
Multiple Correlation
The pairwise correlations between variables are displayed in a multiple correlation matrix, which demonstrates how each variable in the dataset is related to the others. The matrix’s values range from -1 to 1, with 1 denoting the highest possible positive correlation, -1 denoting the highest possible negative correlation, and 0 denoting the absence of any correlation. In general, the correlation matrix improves our understanding of the tendencies of the associations between various variables. It reveals which variables have a propensity to move in tandem (positive correlation), move in opposition to one another (negative correlation), or show no link at all (correlation close to zero) (Equation 18):
where:
= the correlation coefficient for y and x1,
= the correlation coefficient for y and x2, and
= the correlation coefficient for x1 and x2.
Land Surface Temperature Validation Methods
Using a variety of methodologies, uncertainties in the satellite-derived LST data can be examined and validated (Schneider et al., 2012; Guillevic et al., 2014). In this work, a traditional methodology was used; it involves directly comparing sensor temperatures measured on the ground with the LST data obtained from satellites (Schneider et al., 2012). The Benin Airport (NiMET) and the University of Benin are the locations of two meteorological stations within the research region that were utilised to validate the satellite-derived LST data. These areas were chosen because they had working weather stations. The validation Landsat thermal picture was obtained on 7 March 2022, and the temperature data from these two ground stations were compared to the actual temperatures observed that day. This comparison was performed to see how well the temperatures obtained from satellite data and the temperatures reported at weather stations on the ground stations agreed.
RESULTS AND DISCUSSION
The analyses presented in this section were carried out based on the objectives of this study. The dynamics of the LULC, the accuracy of the image classification process, the spatiotemporal distribution of the LST, and the quantitative relationships between the LST and surface biophysical parameters represent some of the subjects covered. Many different presentation formats are utilised to display the findings of the various investigations, including maps, statistics tables, charts, and graphs.
Land Use / Land Cover Distribution
The built-up area in the study region rose from 19.66% of the total area in 1990 to 23.40% in 2000, according to the results given in Table 6. It then increased further, reaching 44.38% in 2012 and 61.79% in 2022. This increase can be linked to continued urban development and rehabilitation, which have been important components of policy objectives for successive administrations since the state of Edo was founded in 1991. On the other hand, the percentage of the total area covered by vegetation dropped from 71.08% in 1992 to 33.42% in 2012 and then to 15.92% in 2022. Between 1992 and 2022, the percentage of barren land increased steadily, from 9.25% to 22.29%. Table 7 offers more information on the variations in the land cover over time. The built-up land cover increased to 20.98% of the total area in 2012 before falling to 17.41% in 2022, which represents a change of around 3.74% between 1992 and 2002. Between 1992 and 2002, there was a 6.92% difference in the vegetation cover, with a larger difference between 2002 and 2012 of 30.74%. The year 2022 saw a reduction in the vegetation cover by 17.5%. The spatial distribution of the LULC classes shown in Figure 2 is consistent with the statistical information given in Table 6 and Table 7, further supporting the conclusions drawn from these data. Mission Road, Ring Road/Mission Road, Ikpoba Hill Market, Fabolude, Aighewi, Airport Road, the University of Benin sector, and significant crossroads are only a few of the commercial centres that have an impact on the urbanisation of Benin City. Because property is frequently bought to build dormitories to house students at high rental costs, the University of Benin has contributed to the growth in land values along Ugbowo/New Lagos Road. These results are consistent with earlier research by Odjugo et al. (2015) and Olayiwola and Igbavboa (2014), which suggests that migration from rural to urban areas and rapid population increase are the main drivers of urbanisation in Benin City. This tendency is further fuelled by the rising demand for housing, jobs, education, healthcare, and other services. Additionally, according to Puplampu and Boafo (2021), the city’s growth has led to a reduction in green spaces.
Population growth may have played a role in the dynamics of the city’s LULC, as shown by the increase in Benin City’s population from 689,152 in 1992 to 1,727,169 in 2022. The built-up area steadily rose from 3.74% in 2000 to 17.41% in 2022 as a result of the concentration of economic activity in the city centre. Human activity has severely harmed places such as Ring Road, Mission Road, Akpakpava, Ikpoba Hill Market, Airport Road, and the neighbourhood of the University of Benin. These findings agree with those of Olayiwola and Igbavboa (2014). Given the rise of peri-urban neighbourhoods, population growth may play a part in the dynamics of the city’s LULC. The changes in land use and cover are a reflection of the modifications to the urban environment brought on by the state of Edo’s separation from the former state of Bendel in 1991, which increased the demand for land for housing and public facilities.
Accuracy Assessment of the Classified Images
For the accuracy assessment, a total of 400 sample points were produced using Equation 12. The technique contrasts thematic-data-layer pixels with reference pixels that have well-established class designations. Table 8 provides a summary of the report that the algorithm generated. Built-up (Pa = 93.8%, Ua = 92.9%), bare land (Pa = 91.3%, Ua = 76.3%), and vegetation (Pa = 96.0%, Ua = 98.8%) all had excellent producer accuracy (Pa) and user accuracy (Ua) values for the 1992 LULC classification. In 2022, bare land had a Pa of 96.3% and a Ua of 78.1%, while built-up areas had a Pa of 87.7% and a Ua of 97.8%. Meanwhile, vegetation had a Pa of 83.7 % and a Ua of 77.9%. These levels of accuracy are thought to be adequate. The high overall accuracy of the LULC classification in this study for the years 1992 (95.0%), 2002 (92.2%), 2012 (89.0%), and 2022 (89%) is evidence of the LULC classification’s reliability.
Spatio-temporal Distribution of Land Surface Temperature
The temperature gradient was used to examine and categorise the temperature distribution in the study area. The maps of the distribution of the LST for 1992 and 2002 are shown in Figure 3. Cool islands, or regions with LSTs below 22°C, were typically found close to the boundaries of metropolitan areas and along riparian vegetation. The highest LST values of 26°C and higher were found in Benin City’s most urbanised regions, which are distinguished by congested residential areas, high levels of commercial activity, and impervious landscapes.
Due to increased commercial activity and fast urbanisation in 2002, there was a larger dispersion of places with LSTs exceeding 32°C, centred along more distinct borders. There was a further increase in areas with high LSTs from 2012 to 2022, with high temperatures (>30°C) moving toward the north-east axis of the urban area. In 2020, there was a considerable increase in areas with extremely high LSTs (>32°C), as well as a major decrease in regions with lower LSTs (26°C). The practical outcomes of the government’s urban reconstruction projects included a rise in impermeable surfaces, probable floods from exacerbated runoff, and the establishment of UHIs.
Mean Land Surface Temperature of the Land Cover Types
Statistics on the normalised LST for various LULC categories are shown in Table 9. Between 1992 and 2022, the mean LST values for several categories changed dramatically. In 1992, the mean LST for vegetation was the lowest at 22.90°C, while the mean temperatures in populated regions were 26.69°C and 24.65°C, respectively.
The mean LST values for the different LULC types had risen by the year 2000.
Table 6
Land use / land cover distribution (1992, 2002, 2012, and 2022)
Land use / land cover category |
1992 |
2002 |
2012 |
2022 |
|||||||
Area (ha) |
Area (%) |
Area (ha) |
Area (%) |
Area (ha) |
Area (%) |
Area (ha) |
Area (%) |
||||
Built-up |
14798.97 |
19.66 |
17615.70 |
23.40 |
33399.27 |
44.38 |
46504.08 |
61.79 |
|||
Bare land |
6964.38 |
9.25 |
9358.29 |
12.43 |
16710.21 |
22.20 |
16778.16 |
22.29 |
|||
Vegetation |
53502.48 |
71.08 |
48291.84 |
64.16 |
25156.35 |
33.42 |
11983.59 |
15.92 |
|||
Total |
75265.83 |
100 |
75265.83 |
100 |
75265.83 |
100 |
75265.83 |
100 |
Table 7
LULC changes for Benin City and its environs
LULC category |
1992–2002 |
2002–2012 |
2012–2022 |
|||
Area ( ) |
( ) |
Area ( ) |
( ) |
Area ( ) |
( ) |
|
Built-up |
2816.73 |
3.74 |
15783.57 |
20.98 |
13104.81 |
17.41 |
Bare land |
2393.91 |
3.18 |
7351.92 |
9.77 |
67.95 |
0.09 |
Vegetation |
-5210.64 |
-6.92 |
-23135.49 |
-30.74 |
-13172.76 |
-17.5 |
Table 8
Summarised statistics of the accuracy assessment
Class name |
1992 |
2002 |
2012 |
2022 |
Built-up |
93.8, 92.9 |
87.7, 97.8 |
87.3, 94.1 |
87.7, 97.8 |
Bare land |
91.3, 76.3 |
95.1, 72.8 |
97.2, 78.8 |
96.3, 78.1 |
Vegetation |
96.0, 98.8 |
83.7, 77.9 |
85.6, 91.0 |
83.7, 77.9 |
Overall accuracy |
95.0 |
92.2 |
89.0 |
89.0 |
Kappa statistics ( ) |
89.5 |
85.3 |
83.1 |
80.6 |
*Pa = Producer accuracy; *Ua = User accuracy
Examples include a rise in the mean LST of vegetation from 22.90°C in 1992 to 23.54°C in 2002 and an increase in the mean LST of built-up areas from 26.69°C to 27.74°C. The fact that the mean LST for vegetation was higher in 2022 than it was for bare land (25.77°C vs 24.65°C) suggests that vegetation is changing into other land cover categories. Built-up regions experienced a rise in temperature of 3.97°C, bare land experienced an increase of 3.54°C, and vegetation experienced an increase of 2.87°C over the 30-year research period, according to the mean LST difference between 1992 and 2022 for various LULC categories.
In built-up regions, the LST standard deviation in 2022 was 1.18°C, suggesting large temperature changes in these areas that may be caused by radiation from various materials and impermeable surfaces. With a standard deviation of 1.09°C, the vegetation showed homogeneity in its surface temperature. The urban canopy heat island phenomenon in Benin City was examined by Aruya et al. (2020), who found similar results that corroborate the mean LST trends observed for various land cover types. According to the study, variations in the mean LST may be caused by more human activity, greater emissivity from traffic and vehicle activity, and the surface cover morphology.
The Urban Thermal Field Variance Index Within Benin City
The UTFVI and Equation 12 were used in the study to objectively detect UHIs. For better visualisation, UHI maps were produced, and the land area was classified into the UHI categories none, weak, middle, strong, stronger, and strongest. Table 10 summarises the area extents of the six categories and their percentages of the total area. The ‘none’ category was 26% of the total study area in 1992, and the ‘strongest’ UHI category was 4%. In 2012, the area extent of the ‘weak’ UHI category was 37%. Conversely, only 6% of the study area was part of the ‘strongest’ UHI category.
Another notable feature of the UHI maps shown in Figure 4 is the directional dispersion of UHIs. The distribution of UHIs has evolved over time, with central business districts (CBDs) serving as the main hub for UHIs. The UHIs spread out over the city as they expanded, affecting places like Uwelu, Upper Siloko, and the Erediawa Road axis, which did not experience UHI effects in 1992.
The results of this study are supported by research by Efe and Eyefia (2014) on urban warming in Benin City. They concluded that Benin City indeed experiences a UHI effect, with an average yearly temperature rise of 4.4°C, which affects both the comfort of urban residents and the bioclimatic features of the urban environment.
In line with the findings of this study, which found that UHI effects were more noticeable in the city’s high-density districts, their study also emphasised the large temperature variance within Benin City’s urban environment.
Table 9
Statistical values of the LST according to the LULC type
|
1992 |
|
2002 |
|
2012 |
|
2022 |
|
Mean LST(°C) |
|
LULC type |
Mean
|
SD (±) |
Mean (°C) |
SD (±) |
Mean (°C) |
SD (±) |
Mean (°C) |
SD (±) |
1990–2020 |
|
Built-up |
26.69 |
0.96 |
27.74 |
0.79 |
29.29 |
0.85 |
30.66 |
1.18 |
3.97 |
|
Bare land |
24.65 |
0.68 |
25.82 |
0.56 |
27.47 |
0.77 |
28.19 |
1.06 |
3.54 |
|
Vegetation |
22.90 |
0.70 |
23.54 |
0.67 |
25.57 |
0.98 |
5.77 |
1.09 |
2.87 |
Table 10
Extracted statistics based on the urban thermal field variance index
Class |
1992 |
2002 |
2012 |
2022 |
||||
Area (ha) |
% |
Area (ha) |
% |
Area (ha) |
% |
Area (ha) |
% |
|
None |
20826.81 |
26 |
7787.7 |
10 |
13077.63 |
17 |
5441.58 |
8 |
Weak |
31988.34 |
41 |
27859.86 |
37 |
27678.96 |
37 |
9122.49 |
12 |
Middle |
10215.63 |
13 |
16338.33 |
22 |
13254.39 |
18 |
12235.5 |
16 |
Strong |
6046.38 |
8 |
9053.82 |
12 |
8189.37 |
11 |
19784.43 |
26 |
Stronger |
6537.33 |
8 |
11554.38 |
15 |
8179.2 |
11 |
20227.95 |
27 |
Strongest |
3228.39 |
4 |
2671.74 |
4 |
4886.28 |
6 |
8453.88 |
11 |

Figure 4 – Urban thermal field variance index map of Benin City in (a) 1992, (b) 2002, (c) 2012, and (d) 2022
Land Surface Temperature Validation Using Ground Data
For validation purposes, Table 10 displays the ground data and the derived LST data. Temperature readings from numerous locations throughout the research area are included in the ground data. These observations were made concurrently with or shortly after the collection of satellite LST data. To allow a direct comparison with the appropriate pixels or areas in the LST data acquired from satellites, the ground measurement locations were correctly recognised and located. To enable the precise synchronisation of ground and satellite observations, geolocation accuracy is essential. For instance, the LST retrieved from the satellite image from 4 January 1992 is 31.33°C; there is a difference of 0.13°C between this temperature and the LST from the ground data provided by the Nigerian Meteorological Agency (NIMET) on the same date, which is 31.46°C. Similar to this, the derived LST from the satellite data from 7 March 2022 was 34.21°C, whereas the ground data LST recorded by the Met. Station was 34.73°C, resulting in a difference of 0.52°C. It is clear from a straightforward comparison that there was little difference between the two temperatures.
Quantitative Relationships Among Surface Biophysical Indices and the Land Surface Temperature
The LULC of the study area was represented by a set of biophysical indicators. These biophysical indices are shown in Figures 5–7. Regression analysis was used to examine the connection between land cover types and the LST. In this study, the LST was regressed with the FVC for vegetation cover and the NDBI for bare land / built-up areas. The surface biophysical indices (the NDVI, FVC, and NDBI) from 1992 to 2022 are displayed in Figures 5–7. The NDBI values grew with time, showing the growth of built-up regions as a result of urban development. On the other hand, when impermeable surfaces and materials took the place of green spaces, the FVC values declined.
Analysing Relationships Between the Land Surface Temperature and Land Use / Land Cover
Multiple correlation was employed to examine the connections between the LST and the land cover indices. It focuses on assessing the overall relationship and the strength of association between a dependent variable and a set of independent variables. Multiple correlation is essential for comprehending the overall predictive ability of a group of predictors for an outcome variable and acts as a key part of multiple regression analysis.
Figure 8 shows the correlation matrices for the years 1992, 2002, 2012, and 2022. The correlation coefficients (R) between the LST and FVC in 1992, 2002, 2012, and 2022 are -0.930, -0.913, -0.907, and -0.805, respectively. These high negative correlation coefficients suggest that as the LSTs of the years under consideration increase, the FVC tends to decrease. Conversely, the R values for the LST and NDBI for 1992, 2002, 2012, and 2022 are 0.953, 0.929, 0.862, and 0.750, respectively. These values indicate a very strong positive correlation between the LST and NDBI in Benin City. This means that an increase in the LST automatically triggers an increase in the NDBI and vice versa.
The correlation coefficient for the FVC and NDBI in 1992 is -0.95466, which indicates a strong negative correlation. This suggests that as the FVC increases, the NDBI tends to decrease. The correlation between the FVC and NDBI tends to be highly negative. These correlations have broadened our understanding of the interactions and dependencies between the variables in the dataset. These results are consistent with those of related studies (Monday et al., 2018; Tanutdech and Teerawong, 2020).
Table 11
Ground-pixel-based temperatures
Station |
Data |
Ground/pixel point |
In-situ temperature |
LST |
Difference |
|
X |
Y |
|||||
NIMET (Benin Airport) |
4 January 1992 7 February 2002 3 February 2012 7 March 2022 |
124471.30 |
700178.89 |
31.46°C
32.10°C
32.51°C 33.64°C |
31.33°C
32.21°C
32.53°C 33.85°C |
0.13°C
-0.11°C
-0.02°C 0.21°C |
Met. Station (University of Benin) |
4 January 1992 7 February 2002 3 February 2012 7 March 2022 |
125343.54 |
707680.17 |
-32.12°C
-32.46°C
-33.50°C 34.73°C |
32.26°C
32.50°C
33.43°C 34.21°C |
-0.14°C
-0.04°C
0.07°C 0.52°C |
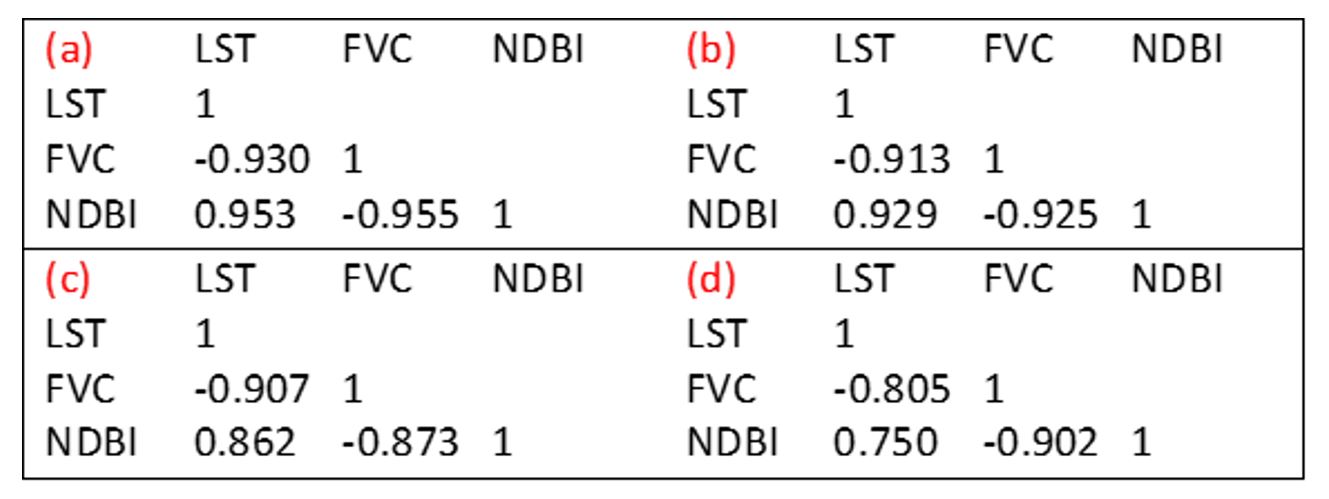
Figure 8 – Multiple correlation coefficients for the LST, FVC, and NDBI in (a) 1992, (b) 2002, (c) 2012, and (d) 2022
CONCLUSIONS
In this study, remote sensing and geographic information systems were utilised to analyse Landsat data to determine the LSTs and various land cover types in Benin City. The dynamics of the LULC between 1990 and 2020 were demonstrated to be influenced by anthropogenic activities. According to this research, between 2000 and 2010, the built-up area increased from 23.40% of the total area to 44.38%. By 2020, the built-up area had climbed to 61.79%. This may be linked to ongoing urban development and restoration, which has been a recurrent theme in the policy frameworks of many administrations since the state of Edo was established in 1991 as a result of the union with the former state of Bendel. This research has helped to improve our understanding of the thermal properties of specific land cover types, such as built-up regions, vegetation, aquatic bodies, and open spaces, and how they contribute to the overall thermal dynamics of a city. Urban planning, climatic mitigation measures, and sustainable development can all benefit from this knowledge. To reduce the UHI effect in Benin City, this study has suggested certain urban land cover measures. This research has offered suggestions for improving urban design components, green infrastructure, and land use policy to lower urban temperatures and improve thermal comfort. This was done by analysing the thermal interactions between various land cover types. The study’s conclusions could aid in the creation of policies and urban-planning decisions that are supported by facts. Urban planners, decision-makers, and stakeholders can use the knowledge collected to learn about the thermal consequences of various land cover types and make more informed choices to build more environmentally friendly and climate-resilient cities.
Author Contributions: OJA wrote the manuscript, OHA went through the data analyses and made corrections, JAO produced the maps, AOO did the proofreading of the manuscript; All authors declared that they had read and approved the publication of the manuscript in this present form.
Funding: There was no external funding for this study.
Acknowledgments: The authors appreciate the contributions of the Forestry Research Institute of Nigeria and thank the Federal University of Agriculture, Abeokuta, Nigeria, for their support in terms of research materials.
Conflicts of Interest: The authors declare no conflict of interest.
REFERENCES
Adam, A.M. Sample Size Determination in Survey Research. Journal of Scientific Research and Reports. 2020, 26, 5, 90-97.https://doi.org/10.9734/jsrr/2020/v26i530263.
Agbor, C.F.; Makinde, E.O. Land Surface Temperature Mapping Using Geoinformation Techniques. Geoinformatics FCE CTU. 2018, 17. https://doi.org/10.14311/gi.17.1.2.
Agheyisi, J.E.; Andrew, O.G. Time Series Analysis of The Spatio – Temporal Indicators of Expansion of Benin City. JORIND. 2013, 11.
Aiyesanmi, A.F.; Imoisi, O.B. Understanding Leaching Behaviour of Landfill Leachate in Benin City, Edo State, Nigeria through Dumpsite Monitoring. British Journal of Environment & Climate Change. 2011, 1, 190-200. https://doi.org/10.9734/BJECC/2011/652.
Akbari, H. Cool Roofs and Pavements to Cool the World: An Integrated Mitigation/Adaptation Strategy for Cities. In Resilient Cities 2011: 2nd World Congress on Cities and Adaptation to Climate Change. Montreal, Canada, 2011.
Akujieze, C.N. Effects of Anthropogenic Activities (Sand Quarrying and Waste Disposal) on Urban Groundwater System and Aquifer Vulnerability Assessment in Benin City, Edo State, Nigeria. PhD Thesis, University of Benin, Benin City, Nigeria, 2004.
Alavipanah, S.; Wegmann, M.; Qureshi, S.; Weng, Q.; Koellner, T. The role of vegetation in mitigating urban land Surface temperatures: A case study of Munich, Germany during the warm season. Sustainability. 2015, 7, 4689-4706. https://doi.org/10.3390/su7044689.
Alzubaidi, L.; Zhang, J.; Humaidi, A.J.; Al-Dujaili, A.; Duan, Y.; Al-Shamma, O.; Santamaría, J.; Fadhel, M.A.; Al-Amidie, M.; Farhan, L. Review of deep learning: Concepts, CNN architectures, challenges, applications, future directions. Journal of Big Data. 2021, 8, 53. https://doi.org/10.1186/s40537-021-00444-8.
Aruya, E.I.; Ariko, J.D.; Ikpe, E. Assessment of Spatial Variation of Urban Canopy Heat Island in The Wet Season in Benin City, Edo State, Nigeria. Gombe Journal of Geography and Environmental Studies (GOJGES). 2020.
Ashley, R.M.; Nowell, R.; Gersonius, B.; Walker, L. Surface Water Management and Urban Green Infrastructure: A Review of Potential Benefits and UK and International Practices. Foundation for Water Research, Buckinghamshire, UK, 2011.
Asmala, A.; Shaun, Q. Analysis of Maximum Likelihood Classification on Multispectral Data. Applied Mathematical Sciences. 2012, 6, 6425-6436.
As-syakur, A.R.; Adnyana, W.S.; Arthana, W.; Nuarsa, W. Enhanced Built-Up and Bareness Index (EBBI) for Mapping Built-Up and Bare Land in an Urban Area. Remote Sensing. 2012, 4, 2957-2970. https://doi.org/10.3390/rs4102957.
Badhe, A.; Chang, S. Fast image classification by boosting fuzzy classifier. Neural Networks & Machine Learning. 2016, 327, 175-182.
Blanusa, T.; Madalena Vaz Monteiro, M.; Fantozzi, F.; Vysini, E.; Li, Y.; Cameron, R.W.F. Alternative to Sedum on Green Roof. Can Broad Leaf Perennial Plants Offer Better Cooling Services? Building and Environment. 2013, 59, 99-106. https://doi.org/10.1016/j.buildenv.2012.08.011.
Bottalico, F.; Chirici, G.; Giannetti, F.; DeMarco, A.; Nocentini, S.; Paoletti, E.; Salbitano, F.; Sanesi, G.; Serenelli, C.; Travaglini, D. Air Pollution Removal by Green Infrastructures and Urban Foresta in the City of Florence. Agriculture and Agricultural Science Procedia. 2016, 8, 243-251. https://doi.org/10.1016/j.aaspro.2016.02.099.
Bouhennache, R.; Bouden, T.; Taleb, A.A.; Chaddad, A. Extraction of urban land features from TM Landsat image using the land features index and Tasseled cap transformation. Recent Advances on Electroscience and Computers. 2015, 142-147.
Breiman, L. Random forests. Machine Learning. 2001, 45, 5-32. https://doi.org/10.1023/A:1010933404324.
Chander, G.; Markham, B. Revised Landsat-5 TM Radiometric Calibration Procedures and Post calibration Dynamic Ranges. IEEE Transactions on Geoscience and Remote Sensing. 2003, 41, 2674-2677. https://doi.org/10.1109/TGRS.2003.818464.
Choi, H.; Lee, W.; Byun, W. Determining the Effect of Green Spaces on Urban Heat Distribution Using Satellite Imagery. Asian Journal of Atmospheric Environment. 2012, 6, 127-135. http://dx.doi.org/10.5572/ajae.2012.6.2.127.
Connop, S.; Vandergert, P.; Eisenberg, B.; Collier, M.J. Re-naturing cities using a regionally-focused biodiversity-led multifunctional benefits approach to urban green infrastructure. Environmental Science and Policy. 2016, 62, 99-111. http://dx.doi.org/10.1016/j.envsci.2016.01.013.
Demuzere, M.; Orru, K.; Heidrich, O.; Faehnle, M. Mitigating and Adapting to Climate Change: Multi-functional and multi-scale assessment of green urban infrastructure. Journal of Environmental Management. 2014, 146, 107-115. https://doi.org/10.1016/j.jenvman.2014.07.025.
Dirisu, D.K.; Frimpong, E.O.; Eguaroje, O.E.; Alaga, A.T. Quantifying Land use/cover change of Oredo, Egor, Ikpoba-Okha LGA, Benin City, Edo State, Nigeria from 1987-2013. International Journal of Scientific & Engineering Research. 2015, 6, 180.
Efe, S.I.; Eyefia, O.A. Urban Warming in Benin City, Nigeria. Atmospheric and Climate Sciences. 2014, 4, 241-252. http://dx.doi.org/10.4236/acs.2014.42027.
Elsayed, I.S.M. A Study on the Urban Heat Island of the City of Kuala Lumpur, Malaysia. In IASTED International Conference on Environmental Management and Engineering. Alberta, Canada, 2009.
Eseigbe, J.O.; Ojeifo, M.O. Aspects of Gully Erosion in Benin City, Edo State, Nigeria. Research on Humanities and Social Sciences. 2012, 2, 21-26.
Ezemonye, M.N.; Emeribe, C.N. Flooding and Household Preparedness in Benin City, Nigeria. Mediterranean Journal of Social Sciences. 2014, 5, 547. http://dx.doi.org/10.5901/mjss.2014.v5n1p547.
Feyisa, G.L.; Dons, K.; Meilby, H. Efficiency of parks in mitigating urban heat island effect: An example from Addis Ababa. Landscape and Urban Planning. 2014, 123, 87-95. https://doi.org/10.1016/j.landurbplan.2013.12.008.
Foster, J.; Lowe, A.; Winkelman, S. The Value of Green Infrastructure for Urban Climate Adaptation, Centre for Clean Air Policy: Washington, DC, USA, 2011, pp. 1-35.
Gago, E.J.; Roldan, R.; Pacheco-Torres, R.; Ordonez, J. The city and urban Heat Islands: a review of strategies to mitigate adverse effects. Renewable and Sustainable Energy Reviews. 2013, 25, 749-758. https://doi.org/10.1016/j.rser.2013.05.057.
Gao, Y.N.; Gao, J.F.; Wang, J.; Wang, S.S.; Li, Q.; Zhai, S.H.; Zhou, Y. Estimating the biomass of unevenly distributed aquatic vegetation in a lake using the normalized water-adjusted vegetation index and scale transformation method. Science Total Environment. 2017, 601, 998-1007. http://dx.doi.org/10.1016/j.scitotenv.2017.05.163.
Giannaros, T.M.; Melas, D. Study of the Urban Heat Island in a Coastal Mediterranean City: The Case Study of Thessaloniki, Greece. Atmospheric Research. 2012, 118, 103-120. https://doi.org/10.1016/j.atmosres.2012.06.006.
Giannini, M.B.; Belfiore, O.R.; Parente, C.; Santamaria, R. Land Surface Temperature from Landsat 5 TM images: comparison of different methods using airborne thermal data measurements and comparison with MODIS remote sensing estimates. Agricultural and Forest Meteorology. 2015, 129, 151- 173.
Gitelson, A.A.; Kaufman, Y.J.; Stark, R.; Rundquist, D. Novel algorithms for remote estimation of vegetation fraction. Remote Sensing of Environment. 2002, 80, 76-87. https://doi.org/10.1016/S0034-4257(01)00289-9.
Guillevic, P.C.; Biard, J.C.; Hulley, G.C.; Privette, J.L.; Hook, S.J.; Olioso, A.; Göttsche, F.M.; Radocinski, R.; Román, M.O.; Yu, Y. Validation of land surface temperature products derived from the visible infrared imaging radiometer suite (VIIRS) using ground-based and heritage satellite measurements. Remote Sensing Environment. 2014, 154, 19-37. https://dx.doi.org/10.1016/j.rse.2014.08.013.
Hathway, E.A.; Sharples, S. The interaction of rivers and urban form in mitigating the Urban Heat Island effect: A UK case study. Building and Environment. 2012, 58, 14-22. https://doi.org/10.1016/j.buildenv.2012.06.013.
Haq, S.M.A. Urban Green Spaces and an Integrative Approach to Sustainable Environment. Journal of Environmental Protection. 2011, 2, 601-608. http://dx.doi.org/10.4236/jep.2011.25069.
Igun, E. Analysis and Sustainable Management of Urban Growth’s Impact on Land Surface Temperature in Lagos, Nigeria. Journal of Remote Sensing and GIS. 2017, 6, 212. http://dx.doi.org/10.4172/2469-4134.1000212.
Ihlen, V. Landsat 7 (L7) Data Users Handbook, Version 2.0. Department of the Interior U.S. Geological Survey, Sioux Falls, South Dakota, November 2019a, pp. 1-139.
Ihlen, V. Landsat 8 (L8) Data Users Handbook, Version 5.0. Department of the Interior U.S. Geological Survey, Sioux Falls, South Dakota, November 2019b, pp. 1-106.
Imhoff, M.L.; Zhang, P.; Wolfe, R.E.; Bounoua, L. Remote sensing of the urban heat island effect across biomes in the continental USA. Remote Sensing of Environment. 2010, 114, 504-513. https://doi.org/10.1016/j.rse.2009.10.008.
Kamavisdar, P.; Saluja, S.; Agrawal, S. A survey on image classification approaches and techniques. International Journal of Advanced Research in Computer and Communication Engineering. 2013, 2, 1005-1009.
Lillesand, T.M.; Kiefer, R. Remote Sensing Image Interpretation. John Wiley, New York, 2008.
Mikias, B.M.; Ikporukpo, C.O.; Olatubara, C.O. Socio-economic characteristics and utilization of urban green infrastructure in southern Ethiopia. International Journal of Development Research. 2017, 07, 18010-18020.
Monday, O.A.; Edigan, B.I.; Aweh, D.S.; Badmus, I.N. Analysis of Land Use Change in Benin City, Nigeria. Geographical Information System & Global Analysis. 2018, 5, 67-71. https://doi.org/10.5819/ijmecs.2018.05.09.
Myint, S.W.; Gober, P.; Brazel, A.; Grossman-Clarke, S.; Weng, Q. Per-pixel vs. object-based classification of urban land cover extraction using high spatial resolution imagery. Remote Sensing of Environment. 2011, 115, 1145-1161. https://doi.org/10.1016/j.rse.2010.12.017.
Nastaran, S. The Impact of Green Areas on Mitigating Urban Heat Island Effect. The International Journal of Environmental Sustainability. 2014, 9, 119-130.
Naushad, R.; Kaur, T.; Ghaderpour, E. Deep Transfer Learning for Land Use and Land Cover Classification: A Comparative Study. Sensors. 2021, 21, 8083. https://doi.org/10.3390/s21238083.
Norton, B.A.; Coutts, A.M.; Livesley, S.J.; Harris, R.J.; Hunter, A.M.; William, N.S.G. Planning for Cooler Cities: A framework to prioritise green infrastructure to mitigate high temperature in urban landscape. Landscape and Urban planning. 2015, 134, 127-138. https://doi.org/10.1016/j.landurbplan.2014.10.018.
Odemerho, F.O. Benin City: A Case Study of Urban Flood Problems. Environmental Issues and Management in Nigerian Development, Evans Brothers, Ibadan, 1988, pp. 280-281.
Odjugo, P.A.; Enaruvbe, G.O.; Isibor, H.O. Geospatial approach to spatiotemporal pattern of urban growth in Benin City, Nigeria. African Journal of Environmental Science and Technology. 2015, 9, 166-175. https://doi.org/10.5897/AJEST2014.1715.
Okhakhu, P.A. The Significance of Climatic Elements in Planning the Urban Environment of Benin City, Nigeria. Unpublished PhD Thesis. Ekpoma-Nigeria: Department of Geography and Regional Planning, Ambrose Alli University, 2010.
Okhakhu, P.A. Assessment of the Urban Climate of Benin City, Nigeria. Journal of Environment and Earth Science. 2016, 6, 131-143.
Olayiwola, A.M.; Igbavboa, O.E. Land Use Dynamics and Expansion of the Built-Up Area in Benin City, Nigeria. Mediterranean Journal of Social Sciences. 2014, 5, 2506-2515. http://dx.doi.org/10.5901/mjss.2014.v5n20p2506.
Olofssona, P.; Foody, G.M.; Herold, M.; Stehmand, S.V.; Woodcocka, C.E.; Wuldere, M.A. Good practices for estimating area and assessing accuracy of land change. Remote Sensing of Environment. 2013, 148, 42-57. http://dx.doi.org/10.1016/j.rse.2014.02.015.
Onaiwu, D.N. Rural-Urban Integration and Spatial Planning in Edo State, Nigeria. Ghana Journal of Geography. 2021, 13, 50-62. https://dx.doi.org/10.4314/gjg.v13i1.3.
Otukei, J.R.; Blaschke, T. Land cover change assessment using decision trees, support vector machines and maximum likelihood classification algorithms. International Journal of Applied Earth Observation and Geoinformation. 2010, 12, S27-S31. https://doi.org/10.1016/j.jag.2009.11.002.
Pakzad, P.; Osmond, P. Corrigendum for developing a sustainability indicator sets for measuring green infrastructure performance. Procedia – Social and Behavioural Sciences. 2016, 2, 16. http://dx.doi.org/10.1016/j.sbspro.2016.02.001.
Parker, J.; Zingoni de Baro, M.E. Green Infrastructure in the Urban Environment: A Systematic Quantitative Review. Sustainability. 2019, 11, 3182. https://doi.org/10.3390/su11113182.
Puplampu, D.A.; Boafo, Y.A. Exploring the Impacts of Urban Expansion on Green Spaces Availability and Delivery of Ecosystem Services in the Accra Metropolis. Environmental Challenges. 2021, 5, 100283. http://dx.doi.org/10.1016/j.envc.2021.100283.
Rimal, B.; Zhang, L.; Keshtkar, H.; Wang, N.; Lin, Y. Monitoring and Modeling of Spatiotemporal Urban Expansion and Land-Use/Land-Cover Change Using Integrated Markov Chain Cellular Automata Model. ISPRS International Journal of Geo-Information. 2017, 6, 288. https://doi.org/10.3390/ijgi6090288.
Rouse, W.; Haas, R.H.; Deering, D.W. Monitoring Vegetation Systems in the Great Plains with ERTS, Third Earth Resources Technology Satellite-1 Symposium. Volume 1: Technical Presentations, section A, 1974.
Sadeghian, M.M.; Vardanyan, Z. The Benefits of Urban Parks, a Review of Urban Research. Institute of Botany, National. Applied Science. 2013, 2, 231-2378.
Schneider, P.; Ghent, D.; Corlett, G.; Prata, F.; Remedios, J. LST Validation Protocol Earth Observation Science, Space Research Centre, Department of Physics & Astronomy, University of Leicester, 2012.
Sejati, A.W.; Buchoria, I.; Rudiartoa, I. The spatio-temporal trends of urban growth and surface urban heat islands over two decades in the Semarang Metropolitan Region. Sustainable Cities and Society. 2019, 46, 101432. http://dx.doi.org/10.1016/j.scs.2019.101432.
Senanayake, I.P.; Welivitiya, W.D.D.P.; Nadeeka, P.M. Remote Sensing Based Analysis of Urban Heat Islands with Vegetation Cover in Colombo city, Sri Lanka using Landsat-7 ETM+ data. Urban Climate. 2013, 5, 19-35. https://doi.org/10.1016/j.uclim.2013.07.004.
Singh, B.; Singh, B.B. Nutritional evaluation of grasses and top foliage through in vitro system of sheep and goats for silvipasture system. Range management Agroforestry. 2017, 38, 241-248.
Skoulika, F.; Santamouris, M.; Kolokotsa, D.; Boemi, N. On the thermal characteristics and the mitigation potential of a medium size urban park in Athens, Greece. Landscape and Urban Planning. 2014, 123, 73-86. https://doi.org/10.1016/j.landurbplan.2013.11.002.
Sobrino, J.A.; Oltra-Carrió, R.; Jiménez-Mu˜noz, J.C.; Julien, Y.; Sòria, G.; Franch, B.; Mattar, C. Emissivity mapping over urban areas using a classification-based approach: Application to the Dual-use European Security IR Experiment (DESIREX). International Journal of Applied Earth Observation and Geoinformation. 2012, 18, 141-147. https://doi.org/10.1016/j.jag.2012.01.022.
Takeuchi, W.; Hashim, N.; Thet, K.M. Application of Remote Sensing and GIS for Monitoring Urban Heat Island in Kuala Lumpur Metropolitan Area. MAp Asia 2010 & ISG 2010. Kuala Lumpur, 2010.
Tanutdech, R.; Teerawong, L. Model of Relationships between Land Surface Temperature and Urban Built-Up Areas in Mueang Buriram District, Thailand. Polish Journal Environmental Studies. 2020, 29, 3783-3790. https://doi.org/10.15244/pjoes/116384.
Thanh Noi, P.; Kappas, M. Comparison of Random Forest, k-Nearest Neighbor, and Support Vector Machine Classifiers for Land Cover Classification Using Sentinel-2 Imagery. Sensors. 2018, 18, 18. https://doi.org/10.3390/s18010018.
Tomlinson, C.; Chapman, L.; Thornes, J.E.; Baker, C. Remote Sensing Land Surface Temperature for Meteorology and Climatology: A review. Meteorological Application. 2011, 18, 296-306. https://doi.org/10.1002/met.287.
USGS (United States Geological Survey). Earth Explorer. 2022. Retrieved from http://earthexplorer.usgs.gov.
United States Geological Survey. Landsat 9 User Handbook Manual. NASA. URL: https://landsat.gsfc.nasa.gov (accessed August 2020).
Ugur, A.; Gordana, J. Automated Mapping of Land Surface Temperature Using LANDSAT 8 Satellite Data. Journal of Sensors. 2016, 1480307. https://doi.org/10.1155/2016/1480307.
Wu, J. Urban Ecology and Sustainability: The State of the Science and future directions. Landscape and Urban Planning. 2014, 125, 209-221. https://doi.org/10.1016/j.landurbplan.2014.01.018.
Yan, Y.; Xu, X.; Xin, X.; Yang, G.X.; Wang, X.; Yan, R.R.; Chen, B.R. Effect of vegetation coverage on Aeolian dust accumulation in a semiarid steppe of northern China. Catena. 2011, 87, 351-356. http://dx.doi.org/10.1016/j.catena.2011.07.002.
Zhao, H.M.; Chen, X.L. Use of Normalized Difference Bareness Index in Quickly Mapping Bare Areas from TM/ETM+. Proceedings of 2005 IEEE International Geoscience and Remote Sensing Symposium, Seoul, Korea, 2005, 3, 1666-1668. http://dx.doi.org/10.1109/IGARSS.2005.1526319.
Academic Editor: Dr. Iuliana Motrescu
Publisher Note: Regarding jurisdictional assertions in published maps and institutional affiliations ALSE maintain neutrality.
Adedeji Oludare Hakeem, Aigbokhan Oseyomon John, Oladoye Abiodun Olusegun, Oyedepo John Adebayo